Researchers from Trinity College Dublin and the University of Bath recently developed a deep neural network-based model that could assist improve the quality of animations featuring quadruped animals like dogs. The framework they developed was presented at the MIG (Motion, Interaction, and Games) 2021 conference, which showcases some of the most cutting-edge technologies for creating high-quality animations and videogames.
Donal Egan, one of the study’s researchers, told TechXplore, “We were interested in working with non-human data.” They chose dogs since they are probably the easiest animal to acquire data from, the researcher noted.
It’s difficult to make high-quality animations of dogs and other quadruped animals. This is due to the fact that these animals move in a sophisticated manner and have distinct gaits with distinct footfall patterns. Egan and his colleagues aimed to develop a system that would make creating quadruped animations easier, resulting in more believable content for animated videos and video games.
Egan noted that creating animations reproducing quadruped motion using traditional methods such as key-framing, is quite challenging. Hence, they thought it would be advantageous to establish a system that could automatically enhance an initial rough animation, removing the need for a user to handcraft a highly realistic one.
Egan and his colleagues’ current research expands on past work targeted at utilizing deep learning to generate and predict human motions. They employed a big set of motion capture data reflecting the movements of a live dog to accomplish similar results with quadruped motions. Several high-quality and realistic dog animations were created using this data.
“For each of these animations, we were able to automatically create a corresponding ‘bad’ animation with the same context but of a reduced quality, i.e., containing errors and lacking many subtle details of true dog motion,” Donal Egan, one of the researchers who carried out the study, told TechXplore. “We then trained a neural network to learn the difference between these ‘bad’ animations and the high-quality animations.”
The researchers’ neural network learned to enhance dog animations by enhancing their quality and making them more lifelike after being trained on good and bad quality animations. The team reasoned that the initial animations may have been made in a variety of ways at run-time, including key-framing approaches, and hence could not be particularly convincing.
“We showed that it is possible for a neural network to learn how to add the subtle details that make a quadruped animation look more realistic,” Egan said. The applications into which our work could be implemented are the practical implications of our work. It may, for example, be used to accelerate an animation workflow. Some apps use methods like classical inverse kinematics to create animations, which can result in animations that lack realism; our work could be used as a post-processing step in such cases.
In a series of tests, the researchers discovered that their deep learning method could considerably increase the quality of existing dog animations without changing the semantics or context of the animation. Their methodology could be utilized to speed up and simplify the creation of animations for usage in films or video games in the future. Egan and his colleagues intend to continue their research into how to digitally and graphically duplicate dog movements in the future.
“Our group is interested in a wide range of topics, including graphics, animation, machine learning and avatar embodiment in virtual reality,” Egan said. “We want to combine these areas to develop a system for the embodiment of quadrupeds in virtual reality—allowing gamers or actors to become a dog in virtual reality. The work discussed in this article could form part of this system, by helping us to produce realistic quadruped animations in VR.”
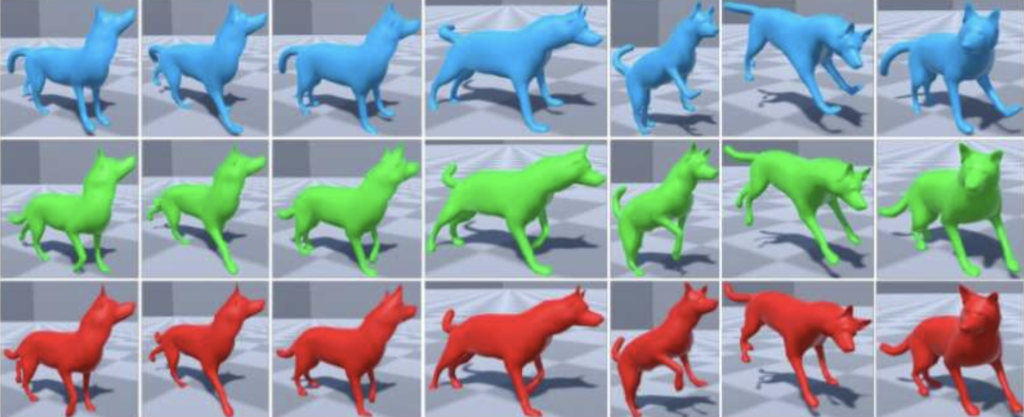
Source: TechXplore