By now, it’s almost old news: big data will transform medicine. It’s essential to remember, however, that data by themselves are useless. To be useful, data must be analyzed, interpreted, and acted on. Thus it is algorithms — not data sets — that will prove transformative. We believe attention therefore has to shift to new statistical tools from the field of machine learning that will be critical for anyone practicing medicine in the 21st century.
First, it’s important to understand what machine learning is not. Most computer-based algorithms in medicine are “expert systems” — rule sets encoding knowledge on a given topic, which are applied to draw conclusions about specific clinical scenarios, such as detecting drug interactions or judging the appropriateness of obtaining radiologic imaging. Expert systems work the way an ideal medical student would: they take general principles about medicine and apply them to new patients.
Machine learning, conversely, approaches problems as a doctor progressing through residency might: by learning rules from data. Starting with patient-level observations, algorithms sift through vast numbers of variables, looking for combinations that reliably predict outcomes. In one sense, this process is similar to that of traditional regression models: there is an outcome, covariates, and a statistical function linking the two. But where machine learning shines is in handling enormous numbers of predictors — sometimes, remarkably, more predictors than observations — and combining them in nonlinear and highly interactive ways.1 This capacity allows us to use new kinds of data, whose sheer volume or complexity would previously have made analyzing them unimaginable.
Consider a chest radiograph. Some radiographic features might predict an important outcome, such as death. In a standard statistical model, we might use the radiograph’s interpretation — “normal,” “atelectasis,” “effusion” — as a variable. But instead, why not let the data speak for themselves? Leveraging dramatic advances in computational power, digital pixel matrices underlying radiographs become millions of individual variables. Algorithms then go to work, clustering pixels into lines and shapes and ultimately learning contours of fracture lines, parenchymal opacities, and more. Even traditional insurance claims data can take on a new life: diagnostic codes trace an intricate, dynamic picture of patients’ medical histories, far richer than the static variables for coexisting conditions used in standard statistical models.
Of course, letting the data speak for themselves can be problematic. Algorithms might “overfit” predictions to spurious correlations in the data; multicollinear, correlated predictors could produce unstable estimates. Either possibility can lead to overly optimistic estimates of model accuracy and exaggerated claims about real-world performance. These concerns are serious and must be addressed by testing models on truly independent validation data sets, from different populations or periods that played no role in model development. In this way, problems in the model-fitting stage, whatever their cause, will show up as poor performance in the validation stage. This principle is so important that in many data science competitions, validation data are released only after teams upload their final algorithms built on another, publicly available data set.
Another key issue is the quantity and quality of input data. Machine learning algorithms are highly “data hungry,” often requiring millions of observations to reach acceptable performance levels.2 In addition, biases in data collection can substantially affect both performance and generalizability. Lactate might be a good predictor of risk of death, for example, but only a small, nonrepresentative sample of patients has their lactate level checked. Private companies spend enormous resources to amass high-quality, unbiased data to feed their algorithms, and existing data in electronic health records (EHRs) or claims databases need careful curation and processing before they are usable.
Finally, machine learning does not solve any of the fundamental problems of causal inference in observational data sets. Algorithms may be good at predicting outcomes, but predictors are not causes.3 The usual common-sense caveats about confusing correlation with causation apply; indeed, they become even more important as researchers begin including millions of variables in statistical models.
Machine learning has become ubiquitous and indispensible for solving complex problems in most sciences. In astronomy, algorithms sift through millions of images from telescope surveys to classify galaxies and find supernovae. In biomedicine, machine learning can predict protein structure and function from genetic sequences and discern optimal diets from patients’ clinical and microbiome profiles. The same methods will open up vast new possibilities in medicine. A striking example: algorithms can read cortical activity directly from the brain, transmitting signals from a paralyzed human’s motor cortex to hand muscles and restoring motor control.4 These advances would have been unimaginable without machine learning to process real-time, high-resolution physiological data.
Increasingly, the ability to transform data into knowledge will disrupt at least three areas of medicine.
First, machine learning will dramatically improve prognosis. Current prognostic models (e.g., the Acute Physiology and Chronic Health Evaluation [APACHE] score and the Sequential Organ Failure Assessment [SOFA] score) are restricted to only a handful of variables, because humans must enter and tally the scores. But data could instead be drawn directly from EHRs or claims databases, allowing models to use thousands of rich predictor variables. Does doing so lead to better predictions? Early evidence from our own ongoing work, using machine learning to predict death in patients with metastatic cancer, provides some indication: we can precisely identify large patient subgroups with mortality rates approaching 100% and others with rates as low as 10%. Predictions are driven by fine-grained information cutting across multiple organ systems: infections, uncontrolled symptoms, wheelchair use, and more. Better estimates could transform advance care planning for patients with serious illnesses, who face many agonizing decisions that depend on duration of survival. We predict that prognostic algorithms will come into use in the next 5 years — although prospective validation will necessarily take several more years of data collection.
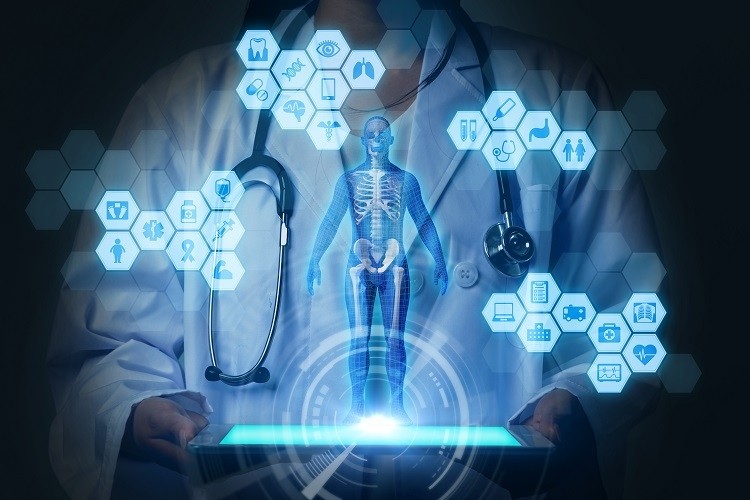
Second, machine learning will displace much of the work of radiologists and anatomical pathologists. These physicians focus largely on interpreting digitized images, which can easily be fed directly to algorithms instead. Massive imaging data sets, combined with recent advances in computer vision, will drive rapid improvements in performance, and machine accuracy will soon exceed that of humans. Indeed, radiology is already part-way there: algorithms can replace a second radiologist reading mammograms5 and will soon exceed human accuracy. The patient-safety movement will increasingly advocate use of algorithms over humans — after all, algorithms need no sleep, and their vigilance is the same at 2 a.m. as at 9 a.m. Algorithms will also monitor and interpret streaming physiological data, replacing aspects of anesthesiology and critical care. The timescale for these disruptions is years, not decades.
Third, machine learning will improve diagnostic accuracy. A recent Institute of Medicine report drew attention to the alarming frequency of diagnostic errors and the lack of interventions to reduce them. Algorithms will soon generate differential diagnoses, suggest high-value tests, and reduce overuse of testing. This disruption will be slower to develop, over the next decade, for three reasons: first, the gold standard for diagnosis is unclear in many conditions (e.g., sepsis, rheumatoid arthritis) — unlike binary judgments in radiology or pathology (e.g., malignant or benign) — making it harder to train algorithms. Second, high-value EHR data are often stored in unstructured formats that are inaccessible to algorithms without layers of preprocessing. Finally, models need to be built and validated individually for each diagnosis.
Clinical medicine has always required doctors to handle enormous amounts of data, from macro-level physiology and behavior to laboratory and imaging studies and, increasingly, “-omic” data. The ability to manage this complexity has always set good doctors apart. Machine learning will become an indispensable tool for clinicians seeking to truly understand their patients. As patients’ conditions and medical technologies become more complex, its role will continue to grow, and clinical medicine will be challenged to grow with it. As in other industries, this challenge will create winners and losers in medicine. But we are optimistic that patients, who generously — if unknowingly — donate the data underlying algorithms, will ultimately emerge as the biggest winners as machine learning transforms clinical medicine.
Published by
Ziad Obermeyer
Ezekiel J. Emanuel
Original