Using electron density, a machine-learning algorithm predicts material properties.
A team lead by scientists at DeepMind, a London-based artificial-intelligence company, has developed a machine-learning model that predicts the distribution of electrons within a molecule to infer its properties. The method, which was published in Science1 on December 10th, can calculate the properties of particular molecules more precisely than existing procedures.
“To make it as accurate as they have done is a feat,” says Anatole von Lilienfeld, a materials scientist at the University of Vienna.
Katarzyna Pernal, a computational chemist from Poland’s Lodz University of Technology, describes the article as “a solid piece of work.” However, she points out that the machine-learning model still has a long way to go before becoming relevant to computational chemists.
Predicting Properties
In principle, quantum mechanics, specifically the Schrödinger equation, which governs the behavior of electron wavefunctions, determines the structure of materials and molecules totally. These are the equations that describe the chances of finding a specific electron at a specific location in space. However, because all electrons interact with one another, computing the structure or molecular orbitals from first principles is a computational nightmare that can only be done for the simplest compounds, such as benzene, according to DeepMind physicist James Kirkpatrick.
To get around this challenge, researchers whose work involves identifying or building novel compounds have relied on a set of approaches known as density functional theory (DFT) to forecast the physical properties of molecules for decades. The theory seeks to compute the total distribution of the electrons’ negative electric charge across the molecule rather than modeling individual electrons. Kirkpatrick explains that “DFT looks at the average charge density, therefore it doesn’t know what individual electrons are.” The density can then be used to calculate the majority of a substance’s properties.
DFT has been one of the most commonly used techniques in the physical sciences since its inception in the 1960s: a 2014 research by Nature’s news team discovered that 12 of the top 100 most-cited papers were about DFT. DFT computations are used extensively in modern databases of material properties, such as the Materials Project.
However, the method has flaws and has been known to produce inaccurate findings for certain types of molecules, including those as simple as salt chloride. And, while DFT computations are far more efficient than those based on basic quantum theory, they are still time-consuming and frequently necessitate the use of supercomputers. As a result, theoretical chemists have increasingly begun to experiment with machine learning in the last decade, focusing on properties such as chemical reactivity and heat conductivity.
Ideal problem
The DeepMind team has made the most ambitious attempt to date to use AI to determine electron density, which is the final result of DFT calculations. “It’s kind of the ideal challenge for machine learning: you know the solution but not the formula you want to use,” says Aron Cohen, a theoretical chemist at DeepMind who has long worked on DFT.
The researchers used data from 1,161 valid Schrödinger equation answers to train an artificial neural network. They also hard-wired some of the known principles of physics into the network to boost accuracy. They then put the trained system to the test on a collection of molecules that are frequently used as DFT benchmarks, and the results were astounding, according to von Lilienfeld. “This is the best the community has come up with, and they outperformed it by a significant margin,” he says.
One advantage of machine learning, according to von Lilienfeld, is that, while training the models requires a lot of processing effort, it only has to be done once. Individual predictions may then be made on an ordinary laptop, saving time and money by not having to recalculate everything each time.
DeepMind is sharing its trained system for anyone to use, according to Kirkpatrick and Cohen. The model now only applies to molecules, not crystal structures of materials, but future versions could, according to the authors, work for materials as well.
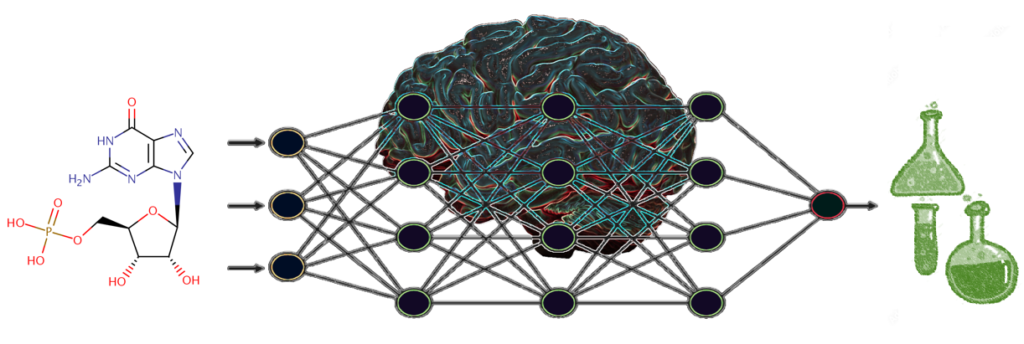
Source: Nature