The difficulty is prioritizing people in all AI efforts. AI experts offer advice on how to create a culture that is both people-centric and AI-driven.
AI is a strong tool for magnifying human knowledge, abilities, and efficiency, as those responsible for developing, building, and deploying AI — from data engineers to developers — appreciate. But how can proponents of AI use AI to improve a stagnant or hostile business culture? That’s definitely the most difficult aspect of AI rollouts.
Entrepreneurs and experts at the forefront of AI revolution understand that this is a problem that technology alone can not fix,”AI cannot solve issues where there are already underlying problems, like a company’s culture or lack of trust from a customer base,” says Stephan Baldwin, founder of Assisted Living Center. “These are fostered by principles that shape the everyday inner and outer workings of a company.”
One of the challenges, Baldwin points out, us “artificial Intelligence models act based on historical data, meaning they’re prone to biases that we humans had when gathering information. Sometimes, an automated process doesn’t take into account the people it governs.”
As a result, the difficulty is to prioritize people in all AI programs. The following are some suggestions from AI experts for creating a people-centered, AI-driven culture:
Extend the IT department’s ownership and responsibilities for AI. AI needs to be a company-wide project including all stakeholders. PROS’s chief AI strategist, Dr. Michael Wu says that “Successful and productive deployment of AI is a cross-functional effort far beyond just data science.” “Extended teams must include IT and cloud operations for security and data governance, as well as change management, training for education, adoption, and best practice on the business side.”
Recognize that AI is nothing more than code. It’s not some enigmatic dark art capable of outwitting people. “AI is no longer magic,” says Beerud Sheth, co-founder, and CEO of Gupshup. Enterprises increasingly seem to comprehend this. “AI is not trying to replace humans but enable a more human-like conversation that has the power of automation and intelligence a machine could have.”
Concentrate AI efforts where they will have the greatest impact. According to Wu, the most important aspects of the business to promote and launch AI differ significantly among industries. However, the underlying theme is that as a by-product of typical business processes, businesses must have a trustworthy source of clean and rich data, he says. Companies with extensive support centers, for example, frequently retain a good operational record of incidents and resolutions because it’s essential for excellent accounting standards, transaction data in sales firms is usually fairly clean. As their AI/ML learns, this data will be used to fuel it. Marketing organizations, on the other hand, have a lot of data as well, but it’s typically noisier and requires cleaning before it can be used.
Customer service, product discovery, and employee-facing areas in customer enterprises experience the highest activity, according to Sheth. “Considerable progress on language parsing and machine learning have enabled fast turnaround time for support queries,” he says. “AI based prediction and context management allow accurate discovery mechanisms to be exposed through simpler interfaces like chats. Machine Learning based cognition engines make query resolution and policy related support issue resolution accurate and easy to deploy on secure channels like MS Teams and progressive web apps.”
Investigate and advocate for the most transformative technology. “Pricing optimization, predictive maintenance, and conversational AI technologies are most impactful because the data required to train and continue to fuel them as they learn tend to be plentiful,” Wu explains. Wu also mentions that their implementation also does not necessitate a significant change in business operations. Furthermore, because there are so many vendors offering these solutions, the total cost of ownership is minimal in comparison to the financial effect these technologies may provide. Multilingual NLP, machine learning, and predictive AI, according to Sheth, have the most potential.
To ensure justice, increase AI’s openness. The conclusions given must be as unbiased and bias-free as possible in order to gain organizational approval and support for AI. Baldwin explains that transparency and fairness are critical for an AI’s success because they build trust by informing both staff and customers about how they are controlled. He also notes that there are countless examples of AI not working correctly, and the last thing you want as a corporation is to be unable to explain why a mistake occurred. According to Wu, more work needs to be done in this area. Many industries that are just beginning to adopt AI are more concerned with getting their AI to work and generating a good ROI with the limited data they have. Fairness isn’t a top focus in these industries, despite the fact that it’s frequently included in corporate narratives. Although everyone preaches about the importance of AI ethics and fairness, not everyone actually does anything about it.
Encourage IT managers and employees to be aware of and trained on fair and actionable AI. Sheth suggests that IT leaders and staff have additional training and awareness to combat AI discrimination. “AI is only as good as the data we feed it.” This is because humans are in charge of the training data. There’s a significant chance our AI systems will be tainted by human prejudice or reflect any other adverse trend that emerges over time. We can develop various models to aid in making better and more equitable decisions, but corporate leaders must also be aware of such difficulties and make the appropriate decisions to assist reduce data bias.
Encourage fair and actionable AI awareness and training at all levels of the enterprise. Although AI is an organizational activity, IT leaders can take the lead in ensuring that AI performs as expected. “Training and education for IT leaders and staff is a good start, but often not sufficient,” says Wu. “Alleviating AI bias should be everyone’s job just like data security, as it’s akin to a company’s business ethics.”
At the same time, he adds, “employees often need to have some incentive to motivate them to exhibit new professional behaviors before they become second nature. These incentives don’t always have to be monetary-related. For example, enterprise gamification can be employed to drive awareness and interest in AI bias mitigation. It can be leveraged within an enterprise to gamify awareness of the AI bias issue, drive positive behaviors that help identify these biases, and even crowdsource for potential solutions.”
A constant examination of AI outputs, according to Sheth, is also required for success. Sheth says that having humans-in-the-loop has been one of the hard-learned lessons for AI firms. He advises frequent assessments of AI findings chosen at random, ensuring that all strata are sufficiently represented in random sampling. End-users may not always have the time or desire to provide feedback on subpar AI results. Evaluate the performance of your models on a regular basis. The feedback from reviewers is transmitted back into the next phase of model training automatically. This method prevents models from becoming obsolete.
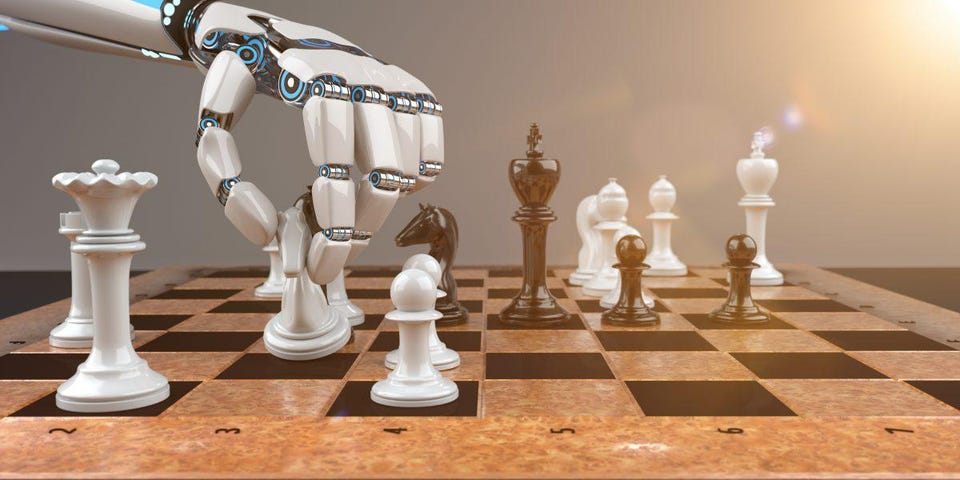
Source: ZDNet