AXA embraces Artificial Intelligence (AI) and Machine Learning (ML) in its business strategy because they provide powerful instruments to create value. This is a worldwide trend, across all industries, more and more processes are managed by these new
technologies.
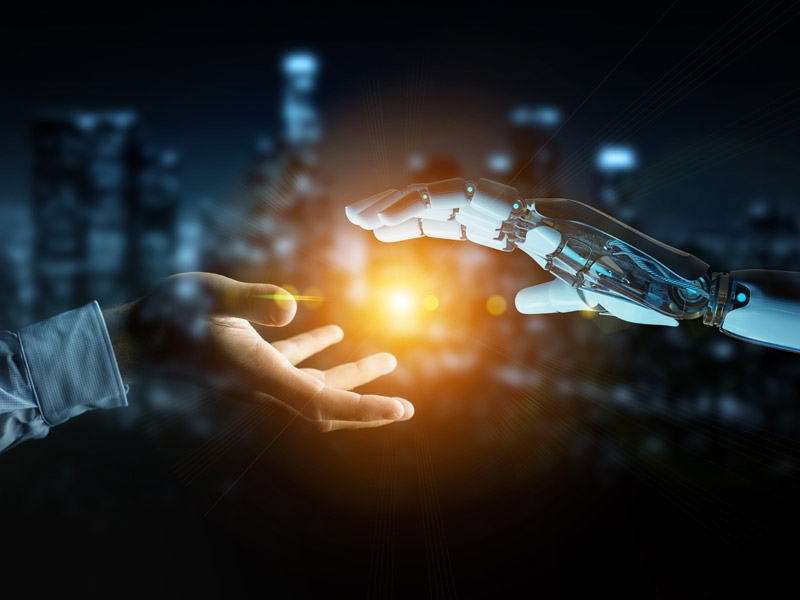
In recent years, Artificial Intelligence (AI) entered a new era. Enabled by an innovative type of algorithms called Machine Learning (ML) algorithms, by the multiplication of data sets and the tenfold increase in processing power, a wide range of applications has emerged, among others automated translation, autonomous car, cancer detection. This gives legitimate rise to hopes for the benefits this new technology will bring to our society.
In this white paper, we identify what we believe are the most fundamental challenges introduced by ML in its intrinsic nature. Other risks, such as misuse of ML, or malfunction resulting from inadequate or unfair input to these algorithms, will not be addressed. Even if those are critical issues, their nature is independent of ML and they are known for a long time. Thus, those cases are already well regulated. For instance, using ML for criminal or intentionally discriminatory purposes, is today covered by criminal or penal law.
As with any new development, besides the great potential of AI, there are also drawbacks associated – some of them yet unexplored. In order to ensure a sustainable success of the AI revolution, it is particularly important to at least roughly understand those risks.
Currently, AXA is fully compliant with the rules set by regulation. Still, we think this is no reason to rest. With this document, we would like to raise awareness of the particular characteristics of ML and stimulate forward-looking actions. Doing so will make our company ready for possible legal changes in the future, and also enable us to continuously follow the ambitious values we have committed ourselves to.
As we will see in the following section, ML algorithms are strongly entangled with data, not only because ML needs data to execute, but also because ML is built, we may even say “grown”, from data.
In most situations, personal data will be used to train the ML algorithm. These data are subject of a special protection, at European level, mainly by the General Data Protection Regulation.
The purpose of this regulation, which entered into force on 25th May 2018, is to harmonize at European level the conditions for the processing of personal data and their use, particularly for decision-making.
In the following, we start by providing some definitions and clarifications around Machine Learning contextual information such as more details on the General Data Protection Regulation. Then, we address three different challenges: fairness and bias, reliability and transparency, and explainability. For each challenge we provide a simplified explanation, followed by the responses provided by regulation today, mainly GDPR.
Based on the current state, we then open the discussion by presenting what we see as limitations of these answers. Our objective is to raise awareness and initiate a fruitful exchange of thoughts in order to anticipate any potential risks well ahead whilst still benefiting from AI. The reader could expect to find recommendations on how to mitigate the above-mentioned risks. We hope that, after reading this document, he or she will fully understand why for each of the challenges presented there is no obvious and simple solution.